How LaaS (Lending as a Service) Is Revolutionizing Fintech
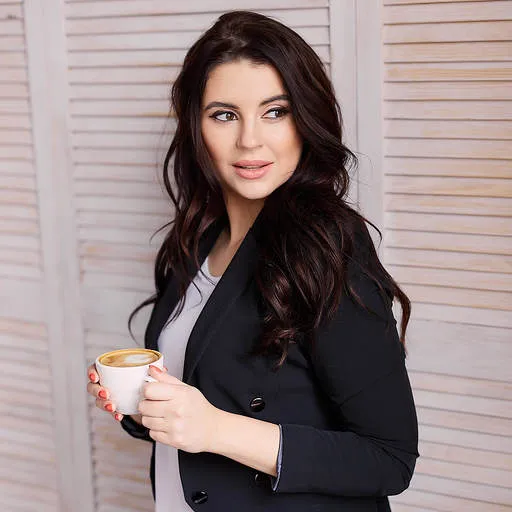

With the never-ending evolution of digital technologies we can see in the last years, businesses can discover more opportunities and options to make a profit, reduce the cost of their operations, and attract/retain customers than ever before. Specifically, this thesis can be applied to the financial industry, one of the leading areas of which is lending.
For example, the independent source Global Data claims that the global digital lending services market will grow at a CAGR of 22.1% until 2030. At the same time, one of the most effective ways of providing such services is by using the Lending as a Service format. Below, we will explain what it is, how such solutions work, what their advantages are, and what impact they can have on the financial sector in the future.
What Is Lending as a Service (LaaS)?
In a nutshell, Lending as a Service is the delegation of all operations related to providing loans to its customers to a third-party provider.
The convenience of SaaS lending technology is obvious even at first glance: instead of independently learning how to manage risks and generally studying how the financial sector and related legislation work, businesses get the opportunity to remain focused on their key operations while expanding their range of services and making them more convenient and accessible to consumers. This approach also reduces the costs associated with the implementation of financial lending services without reducing their effectiveness.
How Lending as a Service Works
From a practical point of view, LaaS is usually presented in the form of cloud solutions that can be adapted to the individual needs of a particular business. Their functionality is available in real time, which means that businesses that have chosen this format of cooperation do not experience any inconveniences associated with delays and downtime.
At the same time, such solutions take on much more than just issuing loans according to the rules and restrictions pre-established by a particular company – they allow these companies to fully automate credit lending services and even speed them up compared to how it could be if they did it personally.
Benefits of Lending as a Service
So, let's formalize the advantages of fintech lending solutions for businesses.
- Less time and overhead expenses. Companies that do not have the opportunity to pay attention to the implementation of lending can significantly benefit from outsourcing loan management. They will also not be forced to deal with tasks related to maintaining their credit platforms since all this will be the responsibility of their suppliers.
- More information about customers. Any average Lending as a Service platform provides businesses with analytical data about their consumers, which can give them valuable insights into their income level, preferences, and other characteristics important for attracting and retaining them. In this way, businesses can significantly boost their efficiency.
- Fewer risks associated with unreliable customers. Typically, the implementation of a lending service is accompanied by the development and deployment of internal Know-Your-Customer (KYC) policies, which may require businesses to become more familiar with how the lending industry works in general and what laws and rules govern it. Conversely, by entrusting all this to a third-party provider, businesses can gain time to solve more pressing problems.
- Wider coverage of the target audience. The rapid implementation of lending services, which LaaS platforms can provide, allows companies to enter new markets and do this much earlier than their competitors. Thus, they gain an increased competitive advantage without the need for a radical modernization of their internal digital infrastructures.
- Accelerated access to advanced IT achievements in the financial sector. Sometimes such a fintech innovation becomes a starting point for more serious updates in the IT infrastructure of a particular business. Indeed, by regularly interacting with LaaS solutions, companies learn more about new digital technologies and the possibilities of their application in a specific business niche, which, in the long term, can give them new ideas for automation and increase the efficiency of their other business operations.
Impact of LaaS on the Financial Industry
It is worth noting that LaaS has an impact not only on individual businesses that have decided to implement such solutions but also on the financial industry as a whole.
Since they actually connect financial institutions (banks), fintech companies, businesses engaged in sale of services/products, and consumers themselves, this interaction chain opens up colossal potential for benefit for each of its links. Indeed: banks get even more clients, companies involved in the development of fintech startups get useful knowledge that allows them to implement standard-compliant solutions, businesses get increased efficiency and adaptability, and consumers themselves get the opportunity to purchase previously unavailable goods and services.
In the long term, all this can lead to the obsolescence of traditional lending models and the opening of new opportunities for cooperation between all the above-described participants in the lending procedure.
Conclusion
If you are considering the digitalization of financial operations in your business and are looking for a reliable service provider providing fintech development services, feel free to contact us. We will create a custom digital banking solution adapted to the standards and generally accepted regulations of your business niche to ensure your company’s sustainability for many years to come.
FAQ
Lending as a Service (LaaS) is when businesses outsource their loan services to a third-party provider. This means that instead of managing loans themselves, companies let an expert handle it, allowing them to focus on their main operations.
LaaS typically uses cloud-based solutions that businesses can customize to fit their needs. These services operate in real-time, enabling faster and automated loan processing without delays.
The benefits of LaaS include reduced costs and time spent managing loans, access to customer insights, reduced risk, the ability to reach new markets quickly, and access to the latest financial technologies.
While LaaS offers many benefits, potential downsides include reliance on third-party providers and the need for strong cybersecurity measures. Businesses should carefully select reputable providers to mitigate these risks.
Related articles

The future of healthcare is digital. In 2022 alone, the global patient portal market reached $2.9B, as the Grand View Research reports. By 2030, it’s projected to grow even higher — at a CAGR of 19.44%. This trend reflects how public and private healthcare organizations strive to digitize paper documentation and automate everyday business operations.
Indeed, the stakes in healthcare are high. Even minor errors can lead to incorrect diagnoses and worsen patients’ conditions. This is where patient portal software greatly minimizes errors, particularly those caused by human factors.
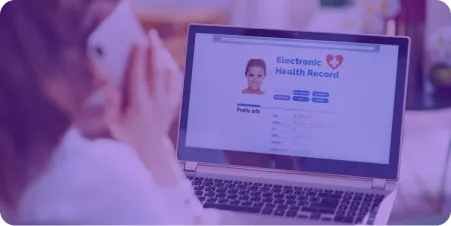
Below, read on how to create a patient portal. We’ll analyze the target audience’s needs, dive into the most successful examples of its implementation, and examine the nature of the target audience's requirements.
What is a patient portal system?
An online patient portal is a web or mobile solution (usually for Android and iOS platforms) that gives patients access to medical records, helps schedule appointments in a few clicks, and easily communicate with healthcare providers at any time.
By automating the interaction between the parties via the patient portal, organizations can improve the quality of care while making their services more accessible and convenient for patients.
In particular, modern patient portal requirements are:
- Fast and secure access to their medical data and records with guaranteed privacy protection.
- High-quality medical services without the need to wait in long queues.
- A clear understanding of the treatment plan and progress.
Healthcare organizations instead aim to:
- Reduce their administrative costs – in particular, for maintaining paper documentation.
- Maximize personnel efficiency without the risk of burnout.
- Increase patient satisfaction through transparent interaction and remote patient monitoring.
- Comply with the healthcare regulations.
- Reach a new level of income by reducing expenses and attracting new patients.
That is why the patient portal system is the one-stop solution that, if implemented correctly, covers all the above-mentioned needs. This is typically achieved through the following features:
- Access to medical records (including medical history and test results).
- Appointment booking (with the option to select the date, time, and doctor).
- Online consultations (for example, via video calls and chats with doctors).
- Repeat prescription orders (automatic issuance of electronic prescriptions and reminders for refills).
- Online bill payment.
- Personal data management (including patient contact information).
- Push notifications (reminders for upcoming visits, tests, vaccinations, etc.).
- Educational materials.
- Feedback tools (surveys for patient satisfaction and forms for submitting complaints, etc.).
Types of patient portals: Which fits your healthcare needs?
Generally, there are two global types of patient portals: integrated and standalone. The former is presented as a component of electronic health records (EMR/EHR) and is fully tied to the infrastructure of a healthcare facility. Integrated portals ensure uninterrupted data exchange between doctors and patients but, in the case of ready-made solutions, are limited by the functionality offered by the specific healthcare provider.

Standalone portals, however, come in two forms: SaaS and on-premise. The former are hosted in the cloud and are available by subscription, which is especially convenient for healthcare providers within small organizations. Their advantage is quick deployment and configuration. At the same time, their customization options are often limited.
As for the second category, these are software solutions installed on local servers of a specific healthcare organization. They provide comprehensive control over data but require significant infrastructure and support costs. They can also be difficult to scale and integrate with third-party solutions.
It is worth noting that every medical organization has its own regular processes and specific business tasks distributed among different departments. In particular:
- The reception desk keeps track of patient records.
- Laboratories collect, process, and store test results.
- The finance department is responsible for payments and insurance processes.
- Doctors and medical personnel work with medical records and appointments.
All the above processes can rarely be integrated into a single system, which makes it difficult to automate them using only one off-the-shelf patient portal. Moreover, medical institutions vary greatly in specialization (including clinics, dentistry, laboratories, hospitals, etc.), and ready-made products do not always meet their needs.
If we add to this the need to comply with regional security standards for patient data, it becomes clear that out-of-the-box solutions may not be viable. This is the main reason why healthcare organizations are increasingly resorting to custom healthcare portal development.
Patient portal requirements: Key features and functionalities
Now, let's check the main functionalities and features that should be integrated into a patient portal.
User authentication and access management system
This portal component uses OAuth 2.0 and OpenID Connect for secure login. It protects users' credentials and enables multi-factor authentication. Usually, developers provide multi-level access with restrictions based on user roles (including patients, doctors, administrators, etc.).
At the same time, when implementing role-based access, it is important to adhere to widely recognized patient data security policies, such as HIPAA and GDPR. Specifically, doctors and administrators should have full access to all patient data. In contrast, individual patients should only be able to view, edit, and delete their info.

Medical data storage
The storage is located on local or cloud servers using AES-256 and TLS 1.3 for data encryption. By the way, many cloud vendors have ready-made solutions for healthcare organizations that meet the standards of patient data privacy (including AWS HealthLake, Azure Healthcare, and others). It is also crucial to ensure that such storage is protected against data misuse and unauthorized access.
Integration with EHR systems
This integration is necessary for consolidating patient data. Thus, developers must adhere to FHIR and HL7 standards to ensure compatibility and data exchange. As for the integration itself, if we are not talking about legacy solutions, it is carried out through an API.
Finally, to ensure that the patient portal system complies with the requirements of the National Coordinator for Health Information Technology (particularly, the ONC's Cures Act), it is essential that the platform could get full access to the patient, with all the ability to edit and delete.
Appointment schedules, doctors’ cards, and telemedicine tools
The first two components involve creating a catalog with therapists’ information and a scheduling system where patients can book visits. This can be achieved through RESTful API to synchronize with hospital calendars. Also, integrating with trusted video communication platforms like Zoom Healthcare can facilitate direct communication between patients and doctors.
Automation of bill calculations and online payments
Online payments are processed through integrations with payment gateways like Stripe or PayPal. In general, they must support PCI DSS standards. As for the automated billing system, this is essentially a regular calculator that “pulls up” data on the cost of services selected by the patient and sums them up (minus insurance and benefits, if any).
Integration with wearable devices
This is necessary so that doctors can monitor the condition of patients in real time. To do this, developers use APIs to collect data from devices (for example, from Apple HealthKit or Google Fit, as well as more specialized IoT equipment). At the same time, they must also implement a mechanism for obtaining prior patient consent to collect personal data.
Messaging
It also makes sense to integrate a chat (with the ability to attach media files) for interaction with doctors, supporting end-to-end encryption (E2E) and using WebSockets protocols that provide real-time messaging.
User dashboards
The patient portal needs an interface for each user role. Basically, it should adapt the data and tools for doctors, admins, and patients. Among other things, it can also be enriched with advanced analytical tools. For example, Power BI or Tableau can be used to generate reports and visualize data (assessing attendance dynamics, treatment results, patient satisfaction, etc.).
How to develop a patient portal software: From concept to launch
When it comes to custom solutions, our telehealth website development services are provided in several subsequent stages. Let’s consider them in detail.
Ideation and requirements analysis
At this stage, our team consolidates the portal concept of custom healthcare portal development, according to your business goals, unique aspects of your internal business processes and IT infrastructure, the needs of the target audience, and an analysis of competitors’ strengths and weaknesses. Based on this blueprint, we create documentation indicating functional and non-functional requirements.
Architecture design
From now on, our patient portal development team has everything ready to define the project's technological stack (for the frontend, backend, and database) and choose a specific architectural approach. We also approve the necessary third-party Integrations (including EHR, payment gateways, telemedicine solutions, etc.).
Finally, we select tools and protocols to ensure our portal has a proper security level, including data encryption, user authentication/authorization methods, diagrams of interaction between components, etc.
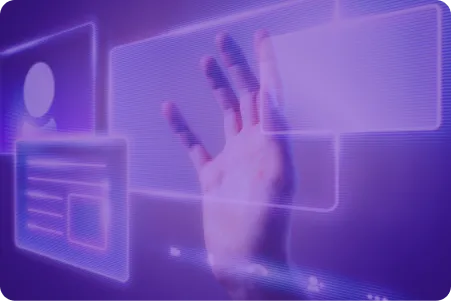
UI/UX design
This stage involves building interface prototypes and gradually transforming them into a full-fledged design after usability testing. At the same time, our patient portal app development experts always take into account the requirements for the accessibility of the user interface. Basically, they are most accurately outlined in the WCAG 2.1 and ADA guidelines.
Development
Now, we can start working on the frontend and backend of the patient portal. Once individual functional modules are ready, we conduct unit testing to prevent hard-to-fix bugs at later stages of the project. This stage also involves the implementation of security mechanisms and integration with third-party solutions.
Testing and quality control
When the code base is ready, our patient portal development experts conduct rigorous testing, including functional, load, security, usability, etc. This stage ensures that the solution meets the best specs and software quality standards in healthcare.
Deployment and launch
Before launching the portal, we prepare all the necessary infrastructure, build a CI/CD pipeline, and set up a monitoring system. If needed, we can train your staff on how to use the portal. After all the previous stages are checked — we usually start deployment.
Post-release support and updates
To better understand its further optimization, our patient portal development experts regularly check the portal’s logs and metrics, collecting feedback. In addition, as post-release bugs are discovered, we fix them and, if necessary, implement new features.
Understanding the needs of your patients
A patient portal and medical website design should fully adapt to the target audience's problems. That is why it is essential to define them in advance. Based on our experience, we would like to highlight the following ones:
- Long wait time when making an appointment.
Patients often encounter long wait times in phone and in-person queues. A patient portal can help. It lets users book online appointments 24/7 and choose a specialist. In addition, it sends SMS or push notifications to remind patients about upcoming visits. - Lack of full access to medical data.
Many patients are forced to visit clinics because healthcare organizations still document their test results manually. The patient portal gives access to electronic medical records, test results, and doctor recommendations, which can be easily downloaded or saved (for offline access). - Lack of understanding of the treatment plans.
Patients may find it difficult to track their treatments' progress, which may result in forgotten, delayed, or canceled visits and procedures. To prevent this, portals display the treatment plan and the current status of therapy. In addition, patients get notifications about upcoming procedures, calendars, and recommendations, which is very smart. - Difficulties in paying for medical services.
The need for patients to be physically present makes offline payments resource-intensive. Patient portals eliminate this headache with integrated payment systems, allowing users to view their payment history and current bills in just a few clicks. - Insufficient communication with the attending physician.
In-person appointments to explain test results and treatment plans can be time-consuming for both patients and healthcare providers. Patient portals streamline this process with online chat and video calling capabilities.

- Weak control over the treatment of chronic conditions.
Patients with chronic conditions often lack convenient tools for regular monitoring. Portals can address this with real-time health data visualization, medication reminders, and appointment scheduling, as well as integrating with wearable devices for continuous data collection. - Low involvement in the treatment process.
Since some patients find it difficult to understand how their actions affect treatment results, the patient portal development team can also add interactive tools to the portal to track patients' treatment progress or provide educational materials.
Gamification elements can enhance the effect.
Patient portal development challenges and solutions
In this section, we will analyze the most typical challenges of patient portal software development and the most effective ways to overcome them.
- Ensuring compliance with regulations.
Telemedicine hugely relies on strong data protection, first — to comply with laws like HIPAA and GDPR, and second — obviously, it is for patient trust. End-to-end encryption, role-based access control (RBAC), and multi-factor authentication are key practices, along with regular system audits and penetration testing to ensure security. - Integration with existing solutions in the organization's IT infrastructure.
Many healthcare providers still use older systems that don't easily connect with modern software. To solve this, developers can build 'middleware' — software that acts as a bridge — to automate data transfer between patient portals and other systems, ensuring everything works smoothly. - Ensuring high performance and scalability.
Many portals frequently freeze when user traffic exceeds the original design capacity. To solve this, developers can host the portal in a cloud environment that scales as users grow and use a microservice architecture to balance the workload across the portal's services. Additionally, they can optimize a database through indexing and caching. - Ensuring intuitiveness.
Since the target audience of patient portals is diverse (including the elderly, people with disabilities, and people with no technical literacy), there should be universal access to the full use of all portal functionality. To solve this, during the development of the patient portal software, the team should conduct user research and surveys to guide and optimize the software effectively. They should also adhere to the compliance of this software with the WCAG 2.1 guidelines.
- Accessibility on mobile OSes.
As patients increasingly manage their healthcare on digital devices, patient portals must offer a seamless mobile experience. Poor mobile optimization can lead to slow loading times and display issues. Developers can address this by building progressive web apps (PWAs) or cross-platform solutions, implementing offline access for key features, and optimizing content loading with techniques like lazy loading and data compression.
Patient portal software cost: What to expect
The cost of telemedicine app development depends on project complexity, the team involved in the patient portal software development process, geographic location, development timeline, and the scope of features. Let's examine each of the points that influenced the cost in detail:
1. Project complexity
- Basic consultation apps with user registration, appointment scheduling, video calls, and messaging: $30K — $100K.
- Advanced telehealth platforms with multi-user portals, integrated EHR, and health analytics: $100K — $300K+.
- Telemedicine platforms with IoT/AI integration: $250K — $500K.
2. Team composition
A typical team for creating a basic patient portal usually includes:
- 2-3 developers: Where 1-2 are front-end developers, and 1 back-end developer.
- The project manager: Tracks the project goals and wins.
- QA specialist: Tests the app to make sure it works correctly.
3. Development timeline
The time it takes for patient portal software development also affects costs. Most telemedicine apps take between 2 to 6 months to develop:
- Simple apps usually take about 2-3 months.
- More complex apps may take up to 6-12 months.
4. Geographic location
American tech experts can be expensive. For example, a front-end developer might earn over $100K annually, about $8K monthly. He might charge between $70K — $80K for a three-month project.
Hiring remote developers can save you money. For instance, Eastern European developers or other regions often charge between $2,500 and $3,500 monthly. You can easily hire a similar team for about $30K to $40K for the same project.
If you want to find out the patient portal software cost in your case, please fill out this contact form.
Improving patient outcomes with data management: A case by Darly Solutions
From theory to practice — let's examine our relevant Darly Solutions experience through a top case from our portfolio.
In particular, we were approached by a HealthTech company specializing in consolidating unstructured medical data for organizations in the healthcare sector. They needed a custom digital solution to monitor clinical data.
Faced with a tight deadline and the need for high precision, we meticulously planned and assigned all the UX/UI design, web development, QA, and project management tasks. We also reviewed the team's work schedule, as some members had to work overtime. Finally, we carefully chose the technology stack. We settled on Angular, HTML, CSS, Docker, ngRx, TailwindCSS, NGX Pagination, and JSON.
Finally, our efforts paid off. We launched the product on time and met all design, functionality, and quality standards. As a result, the client has a competitive product with all the chances to thrive in the innovative telemedicine sector.

Find out more about this case here.
The future of patient portal development
In general, the future of patient portal software development is closely connected with the fast development of modern technologies, such as:
- Artificial intelligence and machine learning.
These technologies will personalize the portal and related healthcare services. AI-powered chatbots, including ones with recognition of human voice commands, will assist with scheduling appointments and answering FAQs. In advance, machine learning can also analyze patient data and symptoms to provide preliminary diagnoses.
Blockchain.
The introduction of blockchain technologies in patient portals can be aimed at more secure storage of their medical records and ensuring access to them only to authorized users. In addition, with its help, each interaction with data (adding, editing, and deleting) will be recorded, increasing the system's transparency.
- Virtual and augmented reality.
These technologies can form the basis of next-generation telemedicine solutions. In particular, in the future, patients will be able to interact with doctors at a distance without reducing the quality of service. AR interfaces will also help inform patients about upcoming treatment plans. - Internet of Things and integration with wearable devices.
IoT solutions can provide end-to-end RPM in real-time (for example, portals will be able to collect and analyze data on the patient's pulse, blood pressure, oxygen level, and activity). Based on this data, doctors can receive instant notifications of critical changes regardless of patients' locations. - Big data and business intelligence.
Examples of patient portals use big data to identify trends, such as tracking the rise in the incidence of a particular virus or assessing the effectiveness of treatments. Also, end-to-end medical data analysis ensures timely prediction of exacerbation of chronic diseases. Finally, doctors and administrators of healthcare institutions will be able to monitor departments' workloads and optimize appointment scheduling. - Biometric identification.
Biometric mechanisms can give users access to the portal through fingerprint, face, or voice scanning, making the authorization process more secure and easier.
Conclusion
Now you know everything about patient portal development and can safely choose an off-the-shelf solution or search for a team to implement a custom one. If the second option is closer to you but you would not like to turn the search process into a real headache, you can contact us, and we will be happy to discuss the details of our future cooperation to implement your idea.
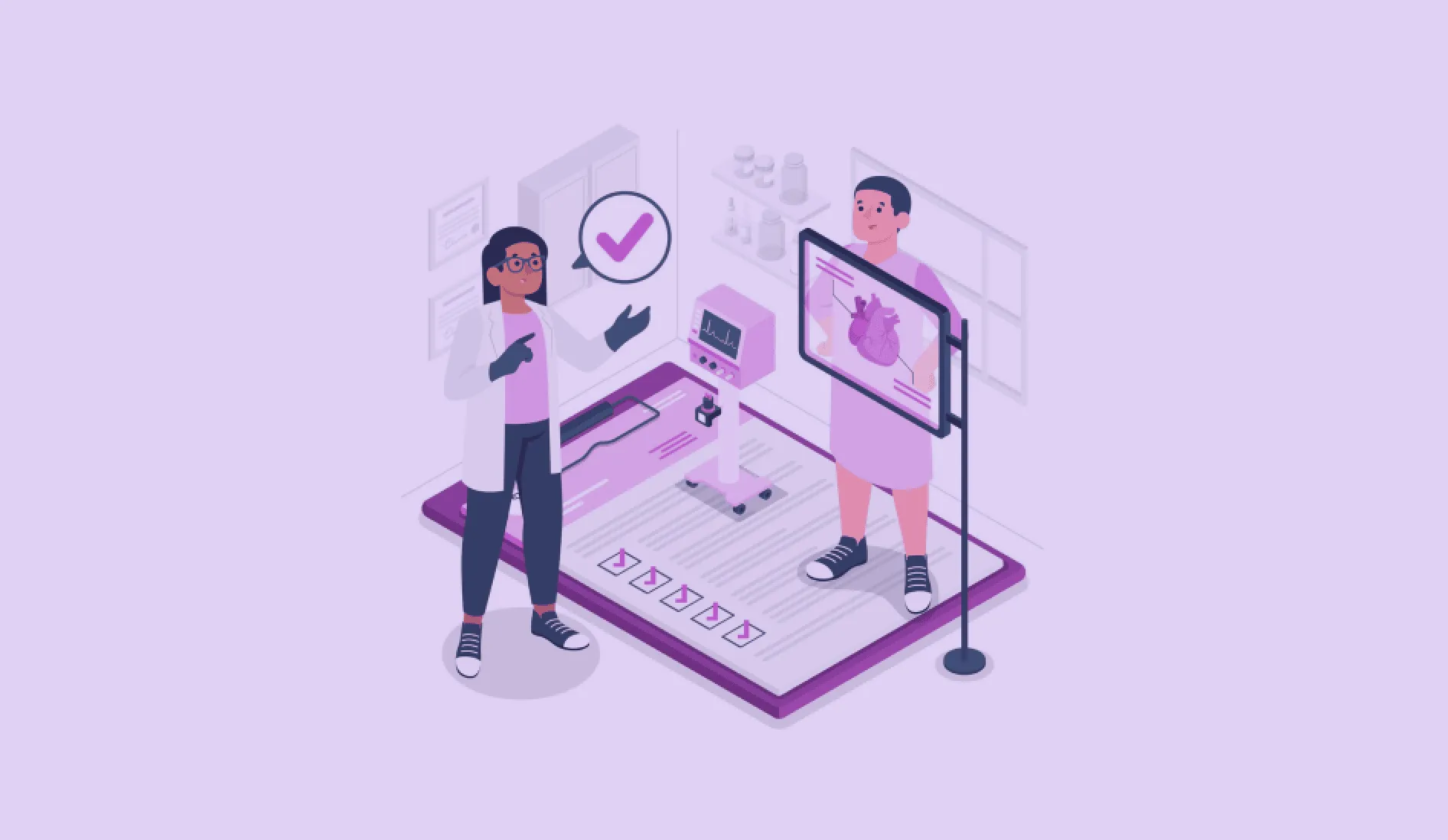
In 2024 alone, the medical imaging software market size reached $8.11B. By 2029, it is projected to grow to $11.83B and up to 7.84% at a CAGR. This is a fairly predictable trend due to the development of AI. Especially since big data, cloud technologies, and other advancements are already significantly speeding up the accuracy of diagnostics.
If you are considering custom development of medical image analysis software, now is the most favorable time. Below, we will reveal the specifics of creating such solutions and describe the requirements and the challenges you may face.
What is the definition of medical imaging software?
Medical imaging software — it's the digital tool doctors use to examine medical images. Think X-rays, MRI and CT scans, ultrasounds, PET, and other radiology scans. Basically, it helps to see the details of every complex illness and make informed decisions about patient care.

To maximize efficiency, medical imaging software integrates a range of advanced technologies. These include AI for anomaly detection, ML for image segmentation, and methods for filtering, contrast enhancement, and noise reduction to improve image quality.
Also, 3D reconstruction technologies create volumetric models of organs and tissues. Developers also rely on the DICOM standard for medical images as it allows seamless transfer. They also use cloud tech to access data, integrated medical records, and VR and AR to visualize data and create interactive interfaces.
As a result, with medical image analysis software, healthcare organizations reduce the workload of their doctors and researchers and minimize the likelihood of misdiagnosis.
Examples of medical imaging software
To better grasp how these solutions work, we suggest you look at several medical imaging software examples that have gained worldwide recognition.
RadiAnt DICOM viewer
It is a high-performance medical imaging software that processes DICOM images. Due to its rich functionality, both doctors and researchers use it in their work. It has smart multimodality tools for 3D and 2D visualization and MPR (multiplanar reconstruction). Moreover, developers made the interface very user-friendly, so this software is also a great choice for users with low technical skills.

OsiriX MD
Specifically designed for macOS, OsiriX MD is a powerful DICOM platform that meets the needs of radiologists. Its advanced capabilities support 3D and 4D image analysis, hybrid imaging with PET-CT and PET-MRI, and integration with PACS servers. Crucially, it is FDA- and CE-certified for clinical use.

Horos
Horos is a free OsiriX-based DICOM viewer available on macOS. It has rich customization options for analyzing volumetric data, such as 3D reconstruction, and is especially useful for students and researchers.
GE Healthcare Centricity PACS
GE Healthcare Centricity PACS is a proprietary enterprise medical image analysis software that analyzes medical images. It has EHR and EMR integration, real-time collaboration, advanced AI analysis, DICOM standards, and format support. It can be a full-fledged assistant for doctors and researchers.

Philips IntelliSpace Portal
Tailored for large clinical institutions, Philips IntelliSpace Portal excels in medical image analysis and visualization. It integrates AI-driven automation and tools for multiparametric imaging in cardiology, neurology, and oncology; this medical imaging software supports multi-user collaboration.
Key features of medical image processing software
This section explores the key functionalities typically found in standard medical imaging software.
Tools for viewing and processing medical images
Ensure your medical imaging software works with various input data (CT scans, MRI scans, X-rays, ultrasounds, and hybrid studies like PET-CT and PET-MRI). Usually, this is done by supporting the DICOM format. In addition, you will need tools to scale, rotate, and adjust image contrast. So, optionally, develop a panel for 3D and 4D visualization, including multiplanar reconstruction.
AI-driven image analysis
AI is key in automating the detection of anomalies in medical scans. It can identify cancerous tumors, blood clots, and fractures early, with a high degree of independence. Also, AI in your medical imaging software can classify pathologies using trained models. It can segment organs and tissues on scans and analyze multiparametric data.
Diagnostic and treatment planning tools
This includes tools for creating 3D models, surgical planning, and evaluating the effectiveness of treatment. You should also consider integrating your medical imaging software with robotic surgical systems.
Medical data management tools
To implement effective medical data management, you will probably need to integrate your medical imaging software with PACS (for storing and transmitting data), EHRs (for centralized access to personal patient information), and cloud solutions (for unimpeded access to images from anywhere in the world where there is an Internet connection).
Collaboration tools
It's mainly for remote access so doctors and specialists can chat and comment on each other's actions. It also involves integrating telemedicine platforms to discuss complex cases and hold educational seminars.
What types of organizations need medical image analysis software development?
A wide range of organizations can benefit from medical image analysis software development. Now, let's find out which areas of healthcare benefit from medical imaging software the most.
- Cardiology.
In this field, medical imaging software is mostly used to analyze CT and MRI of the heart and angiography. In addition, it monitors treatment effectiveness, plans operations, and predicts cardiovascular disease risks.
- Dentistry.
Inevitable for 3D scanning when planning dental implants, diagnosing jaw diseases, visualizing root canals, etc.
- Oncology.
Here, medical imaging software detects and classifies tumors, tracks their growth, and assesses treatment effectiveness.
- Neurology.
In this sector, medical image analysis software analyzes brain MRIs and CTs and provides 3D visualizations to assess the spine and nerves.
- Orthopedics.
Orthopedics studies thrive on precise X-ray analysis, which includes 3D joint modeling and spinal disease diagnostics.
- Mammology.
Medical imaging software can detect microcalcifications and early breast cancer through comparative analysis of changes in mammary gland tissue.
- Urology.
In this industry, medical imaging software helps diagnose kidney and bladder diseases. It does this by analyzing CT and ultrasound images. Additionally, the software can help plan operations and monitor patients with chronic diseases.
- Pulmonology.
Industry specialists can use such software to diagnose lung diseases, analyze chest CT data, and assess COVID-19 damage.
- Gynecology.
In most cases, medical image analysis software is used to perform pregnancy ultrasounds. It helps monitor the fetus, find pelvic tumors, and analyze the endometrium and other tissues.
- Traumatology and emergency medicine.
In traumatology, 3D medical imaging software can quickly diagnose fractures and internal injuries. It can also visualize organs for urgent decisions.

Still, deciding on the right healthcare sector for your medical imaging project? Contact us and discuss the possibilities of its practical implementation with Darly Solutions' experienced developers.
Medical imaging software development: Steps to follow
Custom development must follow clearly defined stages that most teams use. But, it can still be approached in various ways. Below, we outline how healthcare software development services are delivered in our company.
Concept formation
Start your medical imaging software project with market analysis. Define the target audience, prioritize tasks the software should solve, and research competitors (to identify their strengths and weaknesses). Based on the insights, our medical imaging software development team assesses the functional requirements and evaluates the need for specific technologies to handle image processing. This ensures that the chosen solutions align with the project's technical needs and optimize the processing of healthcare-related images.
Planning
Once we agree on the conditions with all stakeholders, we will write a technical specification for your medical imaging software. This document will describe its functionality, interface, API, security, and integration requirements. We will also approve the tech stack and necessary integrations. Finally, we create a roadmap that defines the milestones and deliverables for each medical imaging software development project stage.
Prototyping
Now that everything is ready, we can begin creating user stories. They include handling DICOM file uploads and 3D models, among other key tasks. For UX/UI best practices of safe data, we follow the WCAG 2.1 guidelines. They ensure accessibility for users with varying technical skills. We also test prototypes with focus groups to see feedback on complex features, which is helpful for future design improvement. Finally, after the edits are done, we develop a full-fledged design.
Coding
The frontend has algorithms to process and analyze medical images. The backend ensures secure data transfer between the medical imaging software and storage. It also encrypts data and protects against vulnerabilities like SQL injections. These involve writing database queries for smooth software interactions and data storage interactions. And last but not least — we also integrate with your healthcare org's existing systems and services (if any).
Testing
Once the code for your medical imaging software is ready and all components have passed unit tests, we run complete test cases. We check for load, functional, non-functional, security, and usability issues.

Deployment
At this stage, we are choosing hosting for your medical imaging software (usually either cloud or local servers), setting up CI/CD, and training end users, for example, by providing them with documentation, training materials, or live courses. Once we've done it, we deploy the solution (first in the test environment and then — in the actual usage environment).
Support and updates
Finally, after the medical imaging software is deployed, we set up monitoring systems to track its performance and detect errors, fix post-release bugs, optimize it according to user feedback, and add new features if required.
Key tech specifications for medical imaging software development
Such software development can be complex, especially in its early stages. Basically, there is often no clear way to turn an abstract idea into actual requirements.
So, let's examine all the key tech specifications that are usually implemented in medical imaging software apps:
- Support for common medical image formats such as DICOM (including DICOM tags for metadata) and standards for storing, transmitting, and processing medical images (such as C-STORE, C-FIND, and C-MOVE).
- Compatibility with various devices (CT, MRI, ultrasound, etc.).
- Image processing can improve images by adjusting contrast brightness and applying filters. It can also segment them to highlight organs and tissues. Lastly, it can register them to compare scans over time.
- 2D and 3D visualization, including volume rendering (CT/MRI), support for iso-sections and reconstructions, and interactivity (e.g., rotation, zoom, and pan).
- Data security, including HIPAA and GDPR compliance, support for TLS (for data transfer) and AES-256 (for image and metadata storage) encryption standards, as well as access control with role-based authorization and two-factor authentication.
- PACS and EHR/EMR integration (e.g., via HL7/FHIR).
- Annotation (adding labels, arrows, and text comments) and providing real-time collaboration tools.
- PDF report generation and image export.
- Scalability (including horizontal scaling via the cloud), multi-threading, and hardware acceleration.
- WCAG 2.1 compliance and user interface customization.
- Logging and monitoring events (including loading, processing, and exporting scans), auditing user access, tracking system performance, and setting up failure notifications.
- Local deployment of software on physical servers (most likely, this will require ensuring compatibility with Linux and Windows OS).
- Setting up regular data backups and automatic recovery after system failures.
Of course, this is just a basic list of specifications. In practice, your project team will expand and refine the list of features while specifying the tools and technologies for the project's unique needs.
Medical imaging software development cost
When it comes to the development cost of medical image analysis software it depends on its complexity and the technologies used. Without data and business needs — it's hard to define the precise price, but on average, basic DICOM (Digital Imaging and Communications in Medicine) typically ranges from $30K to $300K. A customized version of Basic DICOM may cost $30K to $50K. Advanced customizations could cost $70K to $150K.
Implementation costs differ based on the size of the practice:
- Small practices typically cost $5K to $10K and take 1 to 2 weeks.
- Medium facilities cost $20K to $50K and take 1 to 3 months.
- Large enterprises may cost $100K to $200K and take 3 to 6 months.
Please complete this form to calculate the precise budget for your medical imaging software development idea. We will contact you shortly.
Challenges in medical imaging software development
Let's examine the main challenges encountered when developing medical imaging software.
- Regulatory compliance.
Software handling sensitive data, like patient information, must comply with HIPAA, GDPR, FDA 21 CFR Part 11, and CE Marking regulations. Key security measures include code audits, RBAC, 2FA, and strong encryption (e.g., AES-256, TLS).
To avoid fines, consult a local lawyer on medical standards.
- Integration with existing systems.
Integrating PACS, EHRs, and other systems requires DICOM, HL7, and FHIR support. Also, medical organizations have very different established IT infrastructures, which makes it hard to unify their software. If you create a universal solution, you must provide some middleware. It will help users adapt to various services and systems.
- High performance and scalability.
Medical images, especially CT and MRI, are large. This can slow their processing and increase resource needs.
In this regard, you may need to implement lossless compression mechanisms for images and multithreading and parallel data processing algorithms. By the way, a common fix is to move your software to a cloud solution designed for healthcare businesses.
- The complexity of big data management.
Storing and processing massive data, like images and metadata, require a careful choice of databases and storage. In particular, this implies a preference for distributed databases and object storage.
For even greater reliability, do not forget to provide backup and auto-recovery.
- Risks associated with cyber attacks.
Cyber attacks that leak medical data are a serious problem for healthcare software. To solve it, you must implement constant monitoring. Also, set up regular security updates, including patches and OS updates.
Finally, train your staff on social engineering. It can reduce the risks of phishing attacks.
Providing a user-friendly interface.
Interfaces for doctors and medical personnel should be user-friendly and intuitive, requiring minimal technical training to operate efficiently. To achieve this goal, you must test hi-fi prototypes on the real target audience and perform subsequent optimizations. Also, do not forget to ensure your interface is created under the WCAG 2.1 guidelines.
The future of medical imaging software
Medical imaging software development will advance by adopting the newest technologies, process optimization, and increased integration with other medical systems.
So, here are the core areas in which medical imaging software can be optimized:
- Speeding up diagnostic.
- Increasing image recognition accuracy.
- Costs reduction.
- Improving user experience.
This can be achieved through the implementation and development of the following technologies:
- Artificial intelligence and machine learning.
For highly accurate and automatic analysis of medical images and accelerated diagnostics.
- Cloud computing.
To provide quick access to medical images from anywhere in the world, process large amounts of data without the need to upgrade local infrastructure, and implement remote collaboration between healthcare specialists.
- VR/AR.
Medical imaging software development allows anatomy and pathologies to be studied using interactive 3D models and visualize the patient's anatomy before surgery.
- Quantum computing.
While most quantum computers are not yet available for widespread use, they will speed up processing large datasets and training neural networks for image recognition in a few years.
- Blockchain.
To guarantee the immutability and protection of data from medical imaging software while providing patients with comprehensive control over their medical information.
Our experience in medical imaging software development
This section covers the development of the PrismaORM brain scanner. This platform was crafted for chiropractors, neurologists, and neurosurgeons to monitor brain activity and brainwaves before, during, and after chiropractic treatments.
First, we assembled a team of eight experts to bring this vision to life. They worked closely with two external teams of medical imaging software engineers. We've pointed out a tech stack based on PostgreSQL, Typescript, React Native, Nest.js, Expo, Three.js, and SQLite. This tech of choice lets us build a platform that processes real-time data from brain activity helmets. The BLE protocol transmits this data. A tablet interface visualizes it. A key to the project's success was optimizing the user experience. This included better platform performance and integrating 3D models.
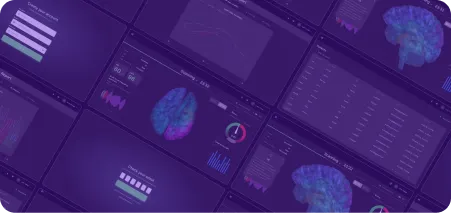
As a result — we've made a powerful tool that empowers medical professionals to conduct more precise diagnostics and offer more effective treatment recommendations.
For a detailed look at this project, visit our portfolio.
Wrapping up
Now that you understand the specifics of medical image analysis software development, you can begin searching for a team to bring your project to life. We are a reliable provider of custom healthtech solutions, ensuring a smooth, transparent, and predictable collaboration. Simply fill out the form, and we'll get in touch as soon as possible to discuss your medical imaging software project in detail.
.webp)
Nowadays, AI technology is significantly changing the different industries and the healthcare industry isn’t an exception. One of the additions – chatbots – has the potential to save time and money.
But should the healthcare industry use a bot service, it is a question many medical workers ask. However, we can define healthcare as a service that happens when patients visit the doctors, we will strictly limit our potential productivity gains. Chatbots can help healthcare providers with customer service and interact with healthcare consumers through text conversationally. So it is one of the most effective solutions to increase patient satisfaction.
According to surveys and researches published by IBM, up to 80% of routine questions could be answered by a chatbot. And it isn’t a surprise that the big part of these questions are asked by the patients and a simple chatbot can help with that.

For being in a win-win situation in healthcare, the providers should think about how to give patients, prospective patients, and other site visitors a way to answer questions, find the important information, and even book appointments as soon as possible without calling in. It’s easier and more convenient for people. It’s more efficient and successful for providers. And it can save a tremendous amount of money and time. That’s the dream, right? In the healthcare sector, the patient’s experience and satisfaction continue to be the top priority.
Chatbots in healthcare can offer the best health care service.
So the real question for clinics, hospitals and other private practices looking to improve the patient experience: Is it worth it?
Will chatbots really help and make a difference for your staff and patients?
At the end of the article you will find the answers to all your questions, so let’s have a look at how healthcare chatbots actually work, their benefits, and potential use cases.
We can divide chatbots into three categories:
Rules-based: Rule-based chatbots can also be named decision-tree bots. This type of chatbot uses a series of defined rules. These rules are the basis for the types of problems the chatbot is familiar with and can deliver solutions for. Usually, there are very simple or complicated rules. They can’t, however, answer any questions outside of the defined rules. These chatbots do not learn through interactions. They only perform and work with the scenarios you train them for. They follow pre-defined, if-this-then-that “playbooks” that automatically respond to a list of pre-populated queries and questions. These chatbots might answer common questions with simple and canned responses. They might also lead people to dead-ends like “I’m having trouble understanding” due to their limited technical capacity.
Adaptive: Using machine learning (ML), adaptive chatbots can learn patterns the more requests they receive.
AI-powered: An AI-powered chatbot is a smarter version that uses natural language processing (NLP) and ML to better understand the intent of the human and provide a more natural, near-human-level communication. An AI chatbot can quickly determine intent and context, move through more complex and nuanced conversations, and deliver the look and feel of chatting with an actual human being.
Chatbots are created to mimic human conversation. Using chatbots, patients can ask health-related questions, check on their appointments, or explore treatment options without calling in.
Why chatbots are perfectly suitable for healthcare
The chatbot is useful for any type of business. But for the healthcare field, they are especially valuable, because chatbots can provide the required information and actions to patients.
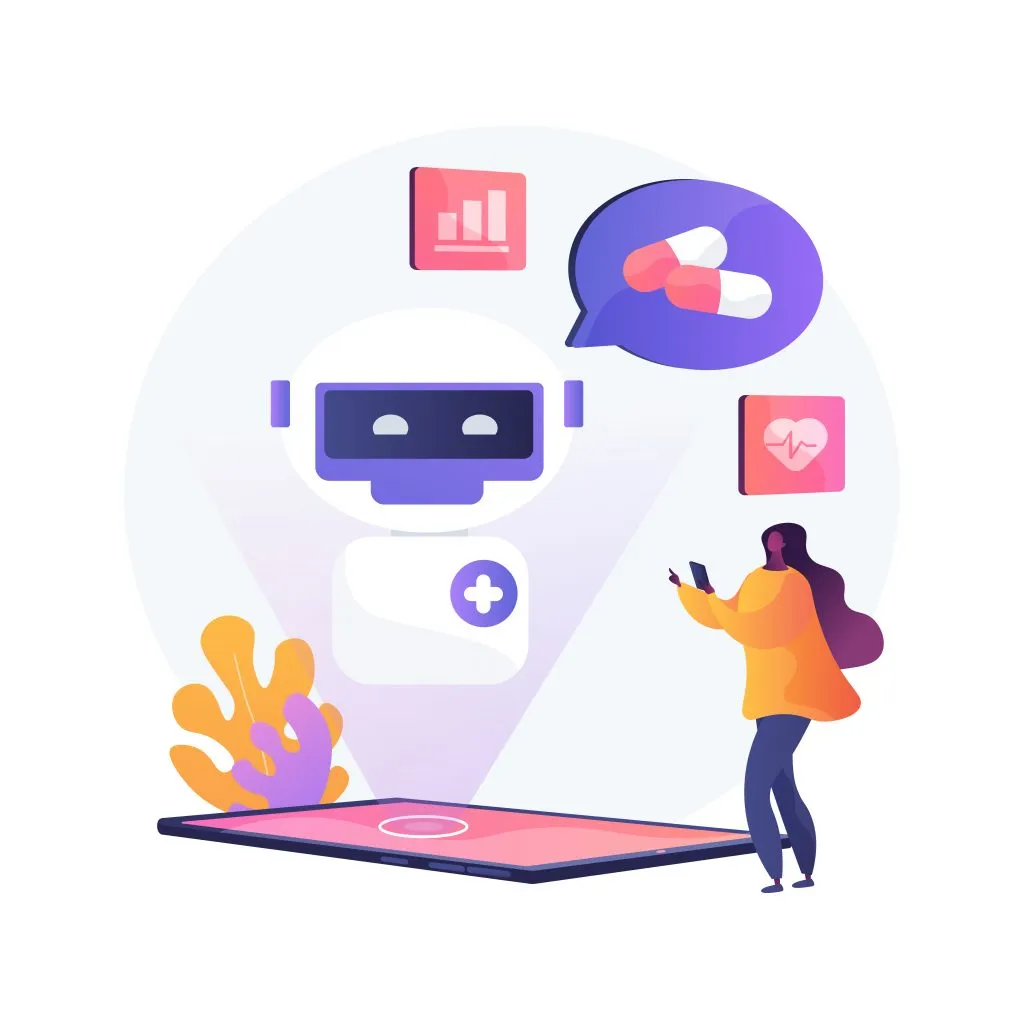
24/7 Service
Anytime chatbots can provide patients service and support. According to this, patients can get immediate engagement and answers.
Follow-up medical care
Quality and results are the most important goals for the patient experience. In many cases, it is difficult for doctors to provide quality care once a patient leaves the hospital. Chatbots could be a good solution for this challenge, and they can give follow-up care by prompting patients to record their symptoms or track their progress.
At-home care
Chatbots can be used anywhere, especially at home. The patient can use the range of actions that include not only looking up symptoms and scheduling appointments, it could also use chatbots for the patients after they are discharged from the hospital. The chatbot could help patients take their medications in time and connect with their doctors.
Keep patient update
This is a new way to address any patient. This will keep the patient and family to remain updated till the last moment. Do you need to go to the appointment? The chatbot will remind you via text message or email. Will the surgery for which you need to be prepared? A chatbot can help patients prepare for any size of surgery or operation beforehand.
Better Patient Experiences
In the end, chatbots no doubt can enhance patient satisfaction. They help improve patient satisfaction scores by providing the convenience of care and reducing wait time. The providers have the opportunity to offer services to the patients and make it convenient for patients to receive medical attention. Chatbots can also facilitate surveys and other patient feedback mechanisms to record and track sentiment.
Use cases in the healthcare industry

- Let’s imagine the patient needs urgent help and information on what to do? The chatbot could quickly quiz users on the health problem they’re facing and recommend a sequence of actions until the ambulance arrives.
- It can be used as a mass email by local or national health organizations. Nowadays, it is especially relevant to people to get to know important details about vaccinations or epidemics.
- It can help patients track their moods or feelings that can be discussed with the psychiatrist or therapist at the next appointment. It could offer tips, notifications and advice between therapy sessions. Furthermore, it even can act more like a personal assistant. It can remind patients to take their medication, help them stay motivated to stick to their schedules and provide other relevant medical information.
- The hospitals and clinics can use the chatbot to allow patients to learn more about their health by asking questions, check symptoms or conditions, and find relevant specialists if they need them.
- The E-commerce industry that sells medical equipment or supplies could build a bot to recommend products to undecided customers. It is one of the ways to increase the visitors on the websites, directing the users to buy the products from it.
- A doctor could set up a chatbot to let users schedule their next appointment easily.
- The bot can be built to help users find in-network providers near them based on city or zip code.
- A medical spa can increase lead generation and user satisfaction using the bot. It is a great idea to ask what users expressly request. Based on the feedback from the users, and add only those services and products which your users have expressly requested. Finally, it could help the best matches schedule a consultation.
Final Thoughts
The chatbots can significantly replace the great part of live chatting with medical personnel. The main advantage that chatbot can provide is improvement in the patient experience. These chatbots are created to be conversational, so they are more responsive and personal than search engines. Chatbots can pull more specific and relevant information for patients rather than search engines. But they can not completely replace a human medical professional’s expertise, but they can reduce the time and money and make it easier for hospital staff to perform their jobs.
Connect with us
.webp)
We are a tech partner that delivers ingenious digital solutions, engineering and vertical services for industry leaders powered by vetted talents.